How to run Mistral 7B on Apple Silicon (M1 and M2)
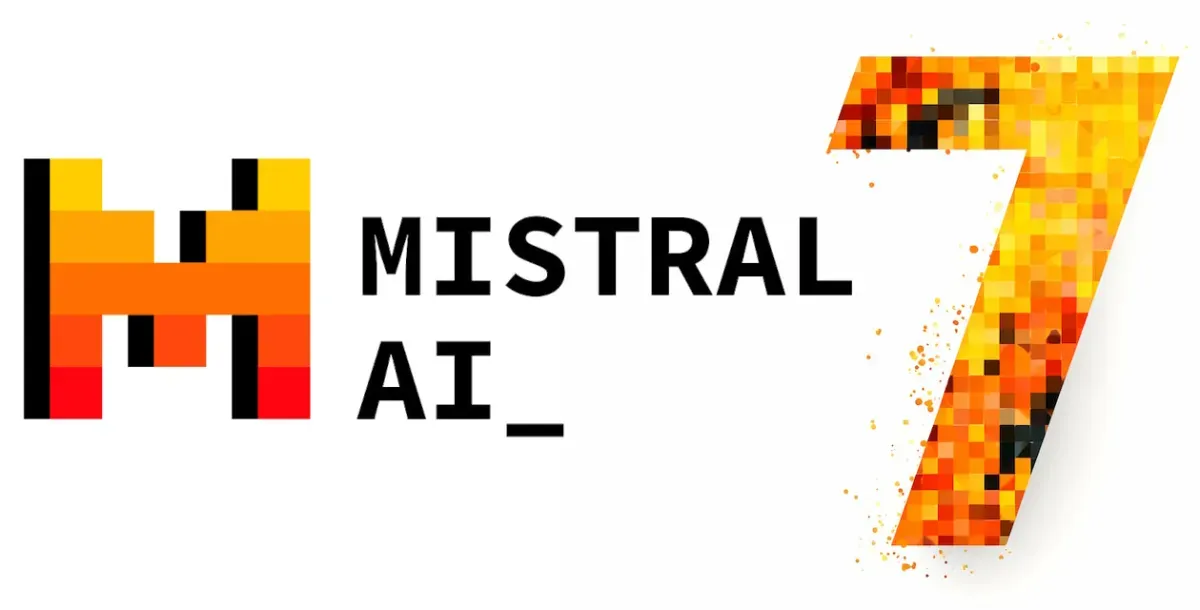
A few days ago, Mistral AI announced their Mistral 7B LLM. This announcement caught my attention for two reasons: 1. Mistral claimed that this model could outperform Llama2 13B with almost half the number of parameters, and 2. this is the first LLM of this quality (that I know of) that is truly "free" for all uses given its release under the Apache 2.0 license.
I decided to get it running on my Mac Studio and was surprised to see that no one had published a "how-to" guide yet. Thankfully, the LLM space is teeming with talented individuals who are passionate about spreading knowledge of this amazing technology so I was able to stitch together a few articles and come up with the end-to-end tutorial below. I hope you find it useful!
For reference, these are the information sources I used to get the model running on my Mac and create this tutorial:
- 4 Steps in Running LLaMA-7B on a M1 MacBook
- TheBloke's Mistral 7B Quantized Models HuggingFace Repository
- llama-cpp-python Mac OS Install Guide
- Open a terminal window and execute the following commands to install prerequisite programs:
xcode-select --install
(Only required if you don't have Xcode installed.)brew install pkgconfig cmake
to install required tools to build the llammacpp source code. (Make sure you have Homebrew installed first.)brew install poetry
to install Poetry. Alternatively, use the official installer.
- Create a directory, cd into it, and initialize Poetry by running the following commands:
mkdir mistral-test
cd mistral-test
poetry init
4.Download the llamacpp source code repository and compile llammacpp.
git clone https://github.com/ggerganov/llama.cpp.git
cd llama.cpp
make
- Install the required Python packages.
poetry add torch torchvision huggingface-hub
- Download a quantized Mistral 7B model from TheBloke's HuggingFace repository. If your Mac has 8 GB RAM, download mistral-7b-instruct-v0.1.Q4_K_M.gguf. For Macs with 16GB+ RAM, download mistral-7b-instruct-v0.1.Q6_K.gguf. (Feel free to experiment with others as you see fit, of course. These are just the ones that make sense to me for each amount of RAM.)
huggingface-cli download TheBloke/Mistral-7B-Instruct-v0.1-GGUF mistral-7b-instruct-v0.1.Q6_K.gguf --local-dir ./models --local-dir-use-symlinks False
(Remember to change the model name according to your RAM amount.)
- Run the model inference.
cd ..
to navigate back up into the llamma.cpp directory../main -m ./models/mistral-7b-instruct-v0.1.Q6_K.gguf -t 8 -n 128 -p 'Q: Who was the first man on the moon? ’
to do a test inference.
- Play around with the model!
- At this point you have a fully working method of talking to the Mistral 7B LLM. Try changing the prompt in the command above and see how it answers!
- [OPTIONAL] Run the model with Python.
cd ..
to change directories back up into the mistral-test directory.poetry add 'llama-cpp-python[server]'
to install the required package that allows us to use llammacpp from Python.- Open your favorite code editor (or notepad if you like to live dangerously) inside the mistral-test directory.
- Create a main.py file, add the following code, and save:
from llama_cpp import Llama
llm = Llama(model_path="./llamma.cpp/models/mistral-7b-instruct-v0.1.Q6_K.gguf")
output = llm("Q: Who was the first man on the moon? A: ", max_tokens=32, stop=["Q:", "\n"], echo=True )
print(output["choices"][0]["text"])
poetry shell
python3 main.py
(If you get missing dependency errors, make sure that your interpreter path points to Poetry's interpreter. You can get this path by runningpoetry show -v
.)
Have fun!
Also, if you want to learn more about Mistral AI, check out this TechCrunch article.